Types of AI Agents
Agents can be grouped into five classes based on their degree of perceived intelligence and capability. All these agents can improve their performance and generate better action over the time. These are given below:
- Simple Reflex Agent
- Model-based reflex agent
- Goal-based agents
- Utility-based agent
- Learning agent
1. Simple Reflex agent:
- The Simple reflex agents are the simplest agents. These agents take decisions on the basis of the current percepts and ignore the rest of the percept history.
- These agents only succeed in the fully observable environment.
- The Simple reflex agent does not consider any part of percepts history during their decision and action process.
- The Simple reflex agent works on Condition-action rule, which means it maps the current state to action. Such as a Room Cleaner agent, it works only if there is dirt in the room.
- Problems for the simple reflex agent design approach:
- They have very limited intelligence
- They do not have knowledge of non-perceptual parts of the current state
- Mostly too big to generate and to store.
- Not adaptive to changes in the environment.
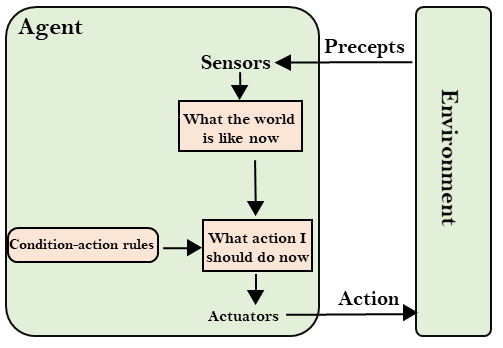
2. Model-based reflex agent
- The Model-based agent can work in a partially observable environment, and track the situation.
- A model-based agent has two important factors:
- Model: It is knowledge about "how things happen in the world," so it is called a Model-based agent.
- Internal State: It is a representation of the current state based on percept history.
- These agents have the model, "which is knowledge of the world" and based on the model they perform actions.
- Updating the agent state requires information about:
- How the world evolves
- How the agent's action affects the world.

3. Goal-based agents
- The knowledge of the current state environment is not always sufficient to decide for an agent to what to do.
- The agent needs to know its goal which describes desirable situations.
- Goal-based agents expand the capabilities of the model-based agent by having the "goal" information.
- They choose an action, so that they can achieve the goal.
- These agents may have to consider a long sequence of possible actions before deciding whether the goal is achieved or not. Such considerations of different scenario are called searching and planning, which makes an agent proactive.
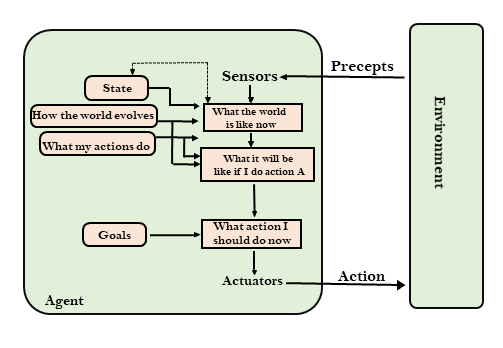
4. Utility-based agents
- These agents are similar to the goal-based agent but provide an extra component of utility measurement which makes them different by providing a measure of success at a given state.
- Utility-based agent act based not only goals but also the best way to achieve the goal.
- The Utility-based agent is useful when there are multiple possible alternatives, and an agent has to choose in order to perform the best action.
- The utility function maps each state to a real number to check how efficiently each action achieves the goals.
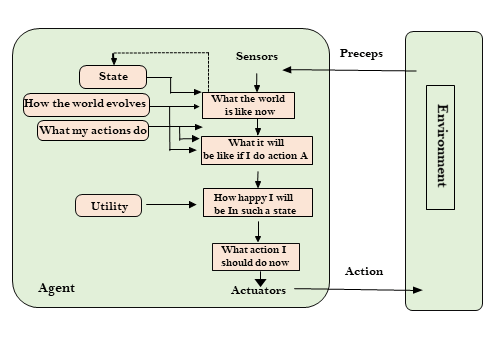
5. Learning Agents
- A learning agent in AI is the type of agent which can learn from its past experiences, or it has learning capabilities.
- It starts to act with basic knowledge and then able to act and adapt automatically through learning.
- A learning agent has mainly four conceptual components, which are:
- Learning element: It is responsible for making improvements by learning from environment
- Critic: Learning element takes feedback from critic which describes that how well the agent is doing with respect to a fixed performance standard.
- Performance element: It is responsible for selecting external action
- Problem generator: This component is responsible for suggesting actions that will lead to new and informative experiences.
- Hence, learning agents are able to learn, analyze performance, and look for new ways to improve the performance.
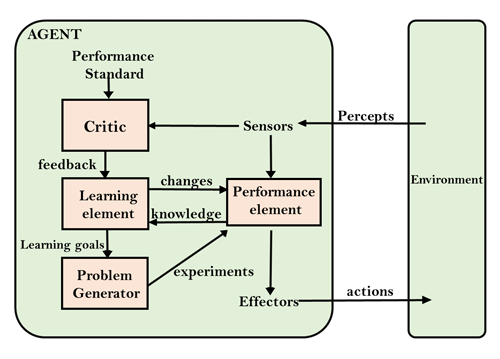
Agents in Artificial Intelligence
An AI system can be defined as the study of the rational agent and its environment. The agents sense the environment through sensors and act on their environment through actuators. An AI agent can have mental properties such as knowledge, belief, intention, etc.
What is an Agent?
An agent can be anything that perceiveits environment through sensors and act upon that environment through actuators. An Agent runs in the cycle of perceiving, thinking, and acting. An agent can be:
- Human-Agent: A human agent has eyes, ears, and other organs which work for sensors and hand, legs, vocal tract work for actuators.
- Robotic Agent: A robotic agent can have cameras, infrared range finder, NLP for sensors and various motors for actuators.
- Software Agent: Software agent can have keystrokes, file contents as sensory input and act on those inputs and display output on the screen.
Hence the world around us is full of agents such as thermostat, cellphone, camera, and even we are also agents.
Before moving forward, we should first know about sensors, effectors, and actuators.
Sensor: Sensor is a device which detects the change in the environment and sends the information to other electronic devices. An agent observes its environment through sensors.
Actuators: Actuators are the component of machines that converts energy into motion. The actuators are only responsible for moving and controlling a system. An actuator can be an electric motor, gears, rails, etc.
Effectors: Effectors are the devices which affect the environment. Effectors can be legs, wheels, arms, fingers, wings, fins, and display screen.
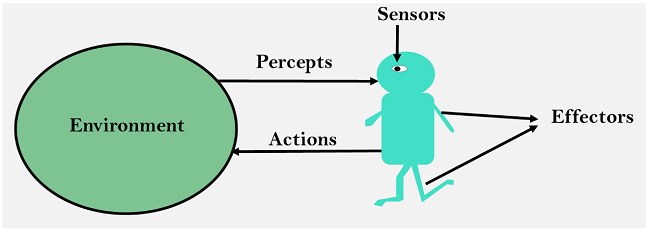
Intelligent Agents:
An intelligent agent is an autonomous entity which act upon an environment using sensors and actuators for achieving goals. An intelligent agent may learn from the environment to achieve their goals. A thermostat is an example of an intelligent agent.
Following are the main four rules for an AI agent:
- Rule 1: An AI agent must have the ability to perceive the environment.
- Rule 2: The observation must be used to make decisions.
- Rule 3: Decision should result in an action.
- Rule 4: The action taken by an AI agent must be a rational action.
Rational Agent:
A rational agent is an agent which has clear preference, models uncertainty, and acts in a way to maximize its performance measure with all possible actions.
A rational agent is said to perform the right things. AI is about creating rational agents to use for game theory and decision theory for various real-world scenarios.
For an AI agent, the rational action is most important because in AI reinforcement learning algorithm, for each best possible action, agent gets the positive reward and for each wrong action, an agent gets a negative reward.
Note: Rational agents in AI are very similar to intelligent agents.
Rationality:
The rationality of an agent is measured by its performance measure. Rationality can be judged on the basis of following points:
- Performance measure which defines the success criterion.
- Agent prior knowledge of its environment.
- Best possible actions that an agent can perform.
- The sequence of percepts.
Note: Rationality differs from Omniscience because an Omniscient agent knows the actual outcome of its action and act accordingly, which is not possible in reality.
Structure of an AI Agent
The task of AI is to design an agent program which implements the agent function. The structure of an intelligent agent is a combination of architecture and agent program. It can be viewed as:
Following are the main three terms involved in the structure of an AI agent:
Architecture: Architecture is machinery that an AI agent executes on.
Agent Function: Agent function is used to map a percept to an action.
Agent program: Agent program is an implementation of agent function. An agent program executes on the physical architecture to produce function f.
PEAS Representation
PEAS is a type of model on which an AI agent works upon. When we define an AI agent or rational agent, then we can group its properties under PEAS representation model. It is made up of four words:
- P: Performance measure
- E: Environment
- A: Actuators
- S: Sensors
Here performance measure is the objective for the success of an agent's behavior.
PEAS for self-driving cars:
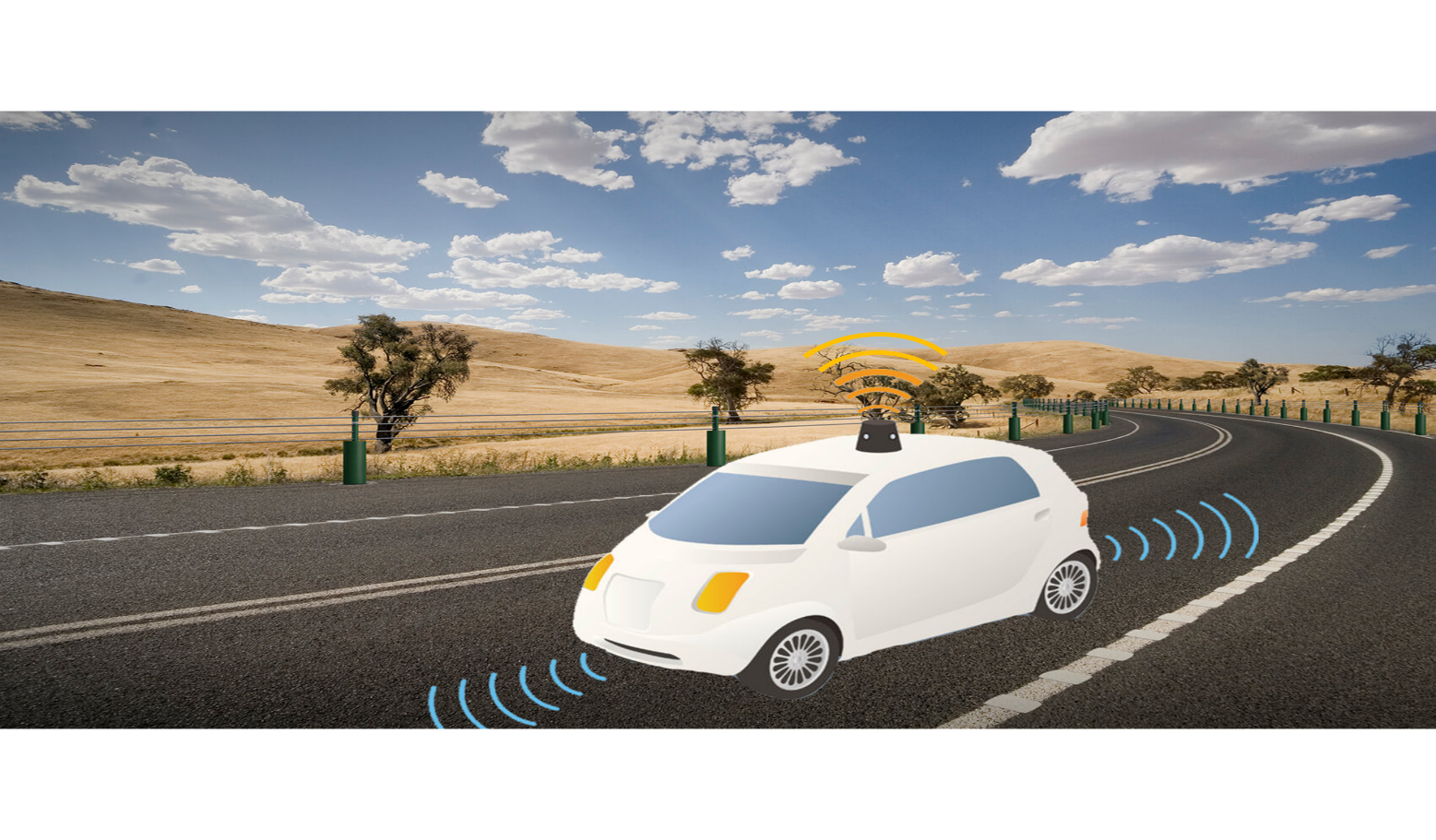
Let's suppose a self-driving car then PEAS representation will be:
Performance: Safety, time, legal drive, comfort
Environment: Roads, other vehicles, road signs, pedestrian
Actuators: Steering, accelerator, brake, signal, horn
Sensors: Camera, GPS, speedometer, odometer, accelerometer, sonar.
Example of Agents with their PEAS representation
Agent | Performance measure | Environment | Actuators | Sensors |
---|---|---|---|---|
1. Medical Diagnose |
|
|
| Keyboard (Entry of symptoms) |
2. Vacuum Cleaner |
|
|
|
|
3. Part -picking Robot |
|
|
|
|
Agent Environment in AI
An environment is everything in the world which surrounds the agent, but it is not a part of an agent itself. An environment can be described as a situation in which an agent is present.
The environment is where agent lives, operate and provide the agent with something to sense and act upon it. An environment is mostly said to be non-feministic.
Features of Environment
As per Russell and Norvig, an environment can have various features from the point of view of an agent:
- Fully observable vs Partially Observable
- Static vs Dynamic
- Discrete vs Continuous
- Deterministic vs Stochastic
- Single-agent vs Multi-agent
- Episodic vs sequential
- Known vs Unknown
- Accessible vs Inaccessible
1. Fully observable vs Partially Observable:
- If an agent sensor can sense or access the complete state of an environment at each point of time then it is a fully observable environment, else it is partially observable.
- A fully observable environment is easy as there is no need to maintain the internal state to keep track history of the world.
- An agent with no sensors in all environments then such an environment is called as unobservable.
2. Deterministic vs Stochastic:
- If an agent's current state and selected action can completely determine the next state of the environment, then such environment is called a deterministic environment.
- A stochastic environment is random in nature and cannot be determined completely by an agent.
- In a deterministic, fully observable environment, agent does not need to worry about uncertainty.
3. Episodic vs Sequential:
- In an episodic environment, there is a series of one-shot actions, and only the current percept is required for the action.
- However, in Sequential environment, an agent requires memory of past actions to determine the next best actions.
4. Single-agent vs Multi-agent
- If only one agent is involved in an environment, and operating by itself then such an environment is called single agent environment.
- However, if multiple agents are operating in an environment, then such an environment is called a multi-agent environment.
- The agent design problems in the multi-agent environment are different from single agent environment.
5. Static vs Dynamic:
- If the environment can change itself while an agent is deliberating then such environment is called a dynamic environment else it is called a static environment.
- Static environments are easy to deal because an agent does not need to continue looking at the world while deciding for an action.
- However for dynamic environment, agents need to keep looking at the world at each action.
- Taxi driving is an example of a dynamic environment whereas Crossword puzzles are an example of a static environment.
6. Discrete vs Continuous:
- If in an environment there are a finite number of percepts and actions that can be performed within it, then such an environment is called a discrete environment else it is called continuous environment.
- A chess gamecomes under discrete environment as there is a finite number of moves that can be performed.
- A self-driving car is an example of a continuous environment.
7. Known vs Unknown
- Known and unknown are not actually a feature of an environment, but it is an agent's state of knowledge to perform an action.
- In a known environment, the results for all actions are known to the agent. While in unknown environment, agent needs to learn how it works in order to perform an action.
- It is quite possible that a known environment to be partially observable and an Unknown environment to be fully observable.
8. Accessible vs Inaccessible
- If an agent can obtain complete and accurate information about the state's environment, then such an environment is called an Accessible environment else it is called inaccessible.
- An empty room whose state can be defined by its temperature is an example of an accessible environment.
- Information about an event on earth is an example of Inaccessible environment.
Comments
Post a Comment